By Nicolas C. Cardenas, Felipe Sanchez, Francisco P. N. Lopes, Gustavo Machado
Published: 13 June 2022
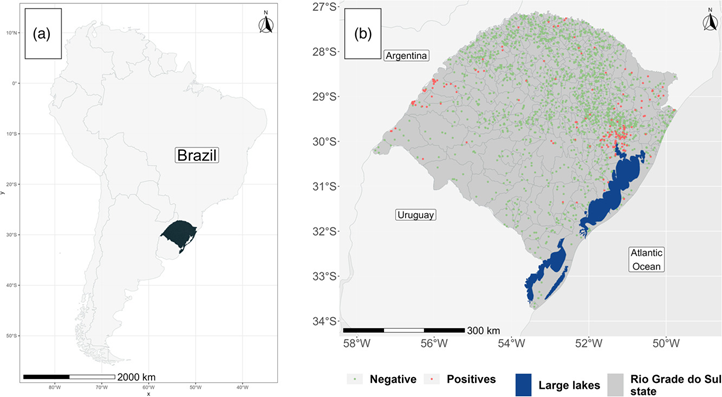
Most animal disease surveillance systems concentrate efforts in blocking transmission pathways and tracing back infected contacts while not considering the risk of transporting animals into areas with elevated disease risk. Here, we use a suite of spatial statistics and social network analysis to characterize animal movement among areas with an estimated distinct risk of disease circulation to ultimately enhance surveillance activities. Our model utilized equine infectious anemia virus (EIAV) outbreaks, between-farm horse movements, and spatial landscape data from 2015 through 2017. We related EIAV occurrence and the movement of horses between farms with climate variables that foster conditions for local disease propagation. We then constructed a spatially explicit model that allows the effect of the climate variables on EIAV occurrence to vary through space (i.e., non-stationary). Our results identified important areas in which in-going movements were more likely to result in EIAV infections and disease propagation. Municipalities were then classified as having high 56 (11.3%), medium 48 (9.66%), and low 393 (79.1%) spatial risk. The majority of the movements were between low-risk areas, altogether representing 68.68% of all animal movements. Meanwhile, 9.48% were within high-risk areas, and 6.20% were within medium-risk areas. Only 5.37% of the animals entering low-risk areas came from high-risk areas. On the other hand, 4.91% of the animals in the high-risk areas came from low- and medium-risk areas. Our results demonstrate that animal movements and spatial risk mapping could be used to make informed decisions before issuing animal movement permits, thus potentially reducing the chances of reintroducing infection into areas of low risk.
More info at: https://onlinelibrary.wiley.com/doi/10.1111/tbed.14627